Understanding Edge AI: Mechanics and Functionality
Recent advancements in AI effectiveness, the widespread use of IoT devices, and the emergence of edge computing have converged to unleash the potential of edge AI, paving the way for unprecedented opportunities. This integration has revolutionized edge AI applications, ranging from aiding radiologists in diagnosing pathologies in medical settings to facilitating autonomous driving and even assisting in agricultural practices like plant pollination.
Numerous analysts and businesses are increasingly exploring and implementing edge computing, a concept rooted in the 1990s with the inception of content delivery networks designed to deliver web and video content efficiently from edge servers located near end-users.
Today, nearly every industry can leverage edge AI to enhance various job functions. Edge applications are propelling the next phase of AI computing, promising to enrich our daily lives at home, work, school, and during transit.
What Exactly Is Edge AI? Edge AI involves deploying AI applications on devices spread throughout the physical world. It’s termed “edge AI” because AI computations occur near users, at the periphery of the network, close to where the data resides, as opposed to centralized processing in cloud facilities or private data centers.
Given the global reach of the internet, the “edge” of the network can denote any physical location, whether it’s a retail outlet, manufacturing plant, hospital, or everyday devices such as traffic lights, autonomous machines, and smartphones.
The Timing of Edge AI Adoption Businesses across all sectors are increasingly embracing automation to enhance processes, efficiency, and safety. To achieve this, computer programs must be capable of recognizing patterns and executing tasks reliably and repeatedly. However, the real world is inherently unstructured, presenting an infinite array of circumstances that cannot be fully encapsulated in programs and rules.
Advancements in edge AI have unlocked opportunities for machines and devices to operate with human-like cognition, learning to perform tasks under diverse circumstances, akin to human adaptability.
The effectiveness of deploying AI models at the edge stems from three key recent innovations:
- Maturation of Neural Networks: Neural networks and related AI infrastructure have advanced to the stage of enabling generalized machine learning, enabling organizations to train and deploy AI models successfully in production environments at the edge.
- Advances in Compute Infrastructure: High-performance distributed computational capabilities are essential for running AI at the edge. Recent developments in highly parallel GPUs have been harnessed to execute neural networks efficiently.
- Adoption of IoT Devices: The widespread adoption of the Internet of Things has led to an explosion of big data. With the ability to collect data from various sources such as industrial sensors, smart cameras, and robots, the necessary data and devices for deploying AI models at the edge are now available. Additionally, the advent of 5G technology has bolstered IoT by providing faster, more stable, and secure connectivity.
Why Opt for Edge AI? What Are Its Advantages? AI algorithms excel at understanding various forms of unstructured information such as language, images, sounds, and more, making them invaluable in environments with real-world problems. These applications would be impractical or impossible to deploy in centralized cloud or enterprise data centers due to concerns regarding latency, bandwidth, and privacy.
The benefits of edge AI include:
- Enhanced Intelligence: AI applications offer greater power and flexibility compared to conventional applications, capable of responding to diverse inputs, including new ones, thanks to neural networks’ adaptability.
- Real-time Insights: Edge technology analyzes data locally, providing real-time responses to users’ needs without delays associated with long-distance communication to centralized servers.
- Cost Reduction: By moving processing power closer to the edge, applications require less internet bandwidth, resulting in significant savings on networking costs.
- Improved Privacy: Edge AI enables the analysis of real-world data without exposing it to human scrutiny, thereby enhancing privacy for individuals whose personal information needs analysis. Local containment of data and uploading only anonymized analysis and insights to the cloud further enhances privacy, simplifying compliance with data regulations.
- High Availability: Decentralization and offline capabilities make edge AI more resilient, ensuring higher availability and reliability for mission-critical AI applications, even in scenarios with limited internet connectivity.
- Continuous Improvement: Edge AI models become more accurate over time as they train on additional data. When faced with data it cannot process accurately, an edge AI application typically uploads it for retraining, leading to continuous enhancement of model accuracy during its operational lifecycle.
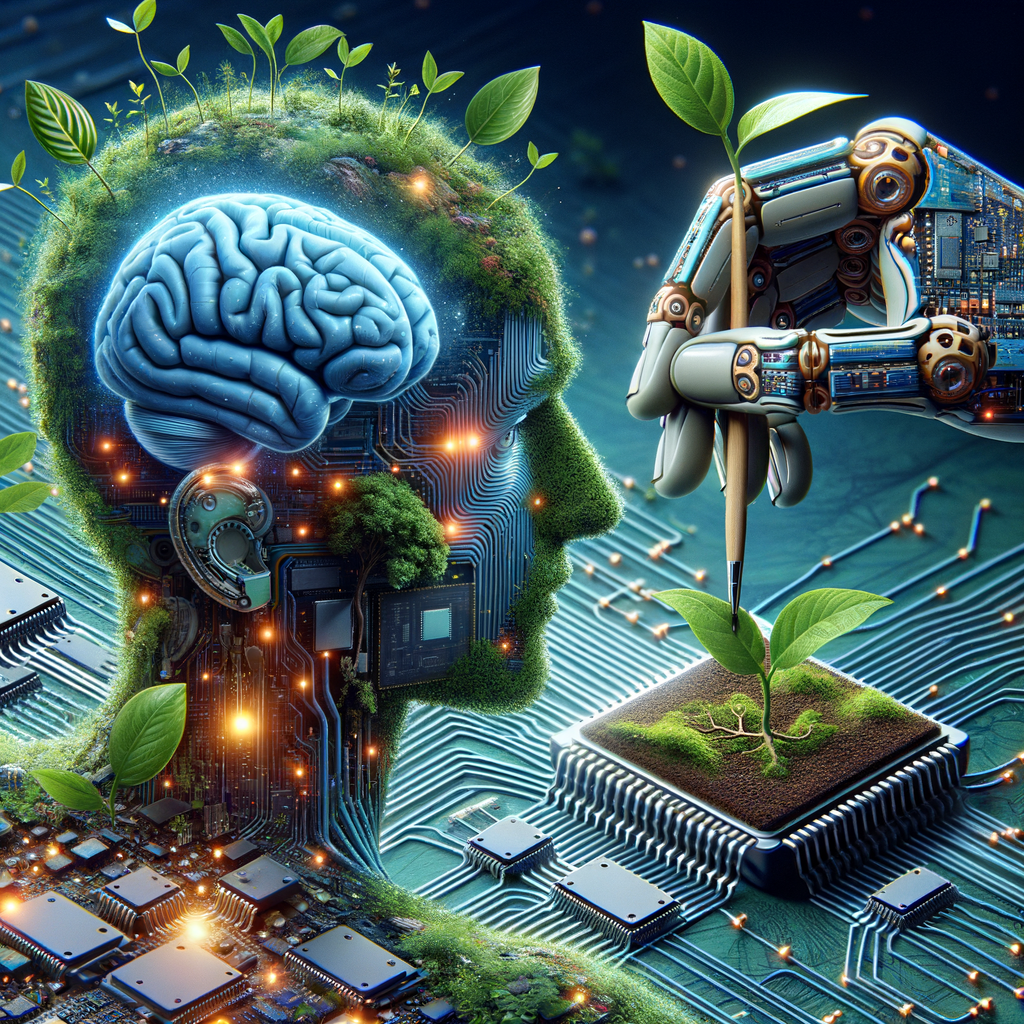
Examples of Edge AI Use Cases AI stands as the most formidable technological force of our era, revolutionizing the world’s major industries. In sectors spanning manufacturing, healthcare, financial services, transportation, energy, and beyond, edge AI is catalyzing novel business outcomes:
- Intelligent Forecasting in Energy: In critical industries like energy, where intermittent supply can jeopardize public welfare, intelligent forecasting is crucial. Edge AI models amalgamate historical data, weather patterns, grid health, and other factors to generate complex simulations. These simulations inform more efficient generation, distribution, and management of energy resources to consumers.
- Predictive Maintenance in Manufacturing: Sensor data facilitates early anomaly detection and prediction of machine failures. Equipment-mounted sensors detect flaws and notify management preemptively, enabling timely repairs and averting costly downtimes.
- AI-Powered Instruments in Healthcare: Cutting-edge medical instruments at the edge are integrating AI capabilities. These devices utilize ultra-low-latency streaming of surgical video to facilitate minimally invasive procedures and provide on-demand insights.
- Smart Virtual Assistants in Retail: Retailers are enhancing the digital shopping experience by introducing voice ordering. Voice commands replace text-based searches, enabling shoppers to effortlessly search for products, access information, and place orders via smart speakers or other intelligent devices.
The Role of Cloud Computing in Edge Computing AI applications can operate either in data centers, such as those in public clouds, or in the field at the network’s edge, near users. Both cloud computing and edge computing offer distinct advantages that can complement each other in deploying edge AI:
Cloud Benefits: Infrastructure cost-effectiveness, scalability, high utilization, resilience against server failure, and collaboration capabilities.
Edge Benefits: Faster response times, reduced bandwidth costs, and resilience against network failure.
Cloud computing supports edge AI deployment in various ways:
- Running the model during training.
- Continuing to run the model during retraining with edge data.
- Supplementing field models with AI inference engines in the cloud for computationally intensive tasks.
- Providing the latest versions of AI models and applications.
- Ensuring consistent deployment of edge AI across device fleets, with cloud-based software management.
The Future of Edge AI With the commercial maturity of neural networks, proliferation of IoT devices, advances in parallel computation, and the advent of 5G, a robust infrastructure for generalized machine learning has emerged. Enterprises now have the opportunity to leverage AI in their operations, leveraging real-time insights while reducing costs and enhancing privacy.
We are merely scratching the surface of edge AI potential, with seemingly endless possibilities awaiting exploration. Organizations can learn how to deploy edge AI by exploring top considerations for deploying AI at the edge.
Creative Virtual AI Assistants
We have trained our AI Chat Bots with the knowledge of industry experts and conversion experts so you can be sure it knows how to do its job and answer all your questions instantly and provide requested information